scOrange Blog
-
Categories:
- clustering
- t-sne
- marker-genes
- tsne
- annotator
- data-mining
- embedding
- embryo
- genomics
- markers
Author: Iva Černoša, Aug 19, 2019
Automatic Embedding of New Cells Onto an Existing Landscape
Learn how to quickly embed new cells onto an existing tSNE projection using the new widget the Annotator on the dataset of a healthy individual and AML patient undergoing chemotherapy gathered by Van Galen et al. (Cell, 2016)
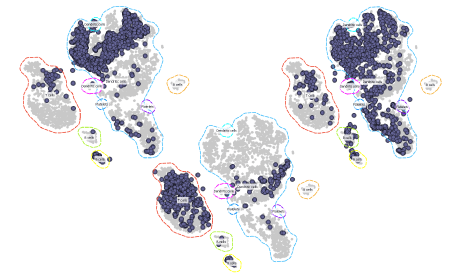
Author: Iva Černoša, May 6, 2019
X And Y Chromosome Expression in Human Preimplantation Embryos
Using the single cell data from Petropoulos et al. (Cell, 2016), we determine the karyotype (XY, XX, X0) of the cells and inspect the time of activation of Y chromosome in a preimplantation embryo.
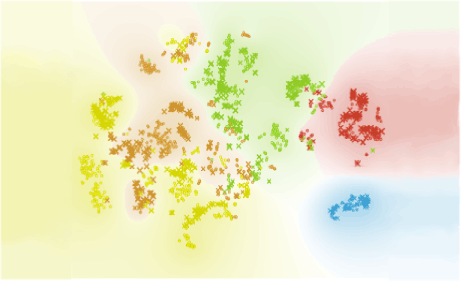
Author: Iva Černoša, Apr 23, 2019
Subsets of Exhausted CD8+ T Cells
We reproduce a part of the study by Miller et al. (Nature Immunology, 2019) through identifying subsets of exhausted CD8+ T cells during chronic viral infections and corresponding groups in T cells isolated from tumours
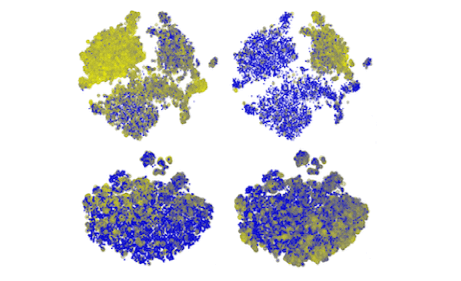
Author: Iva Černoša, Mar 29, 2019
Clustering Cells in Mouse Pancreas
To reproduce a part of a study by Baron et al. (Cell Systems, 2016), we cluster mouse pancreatic cells and identify marker genes for each type of the cells.
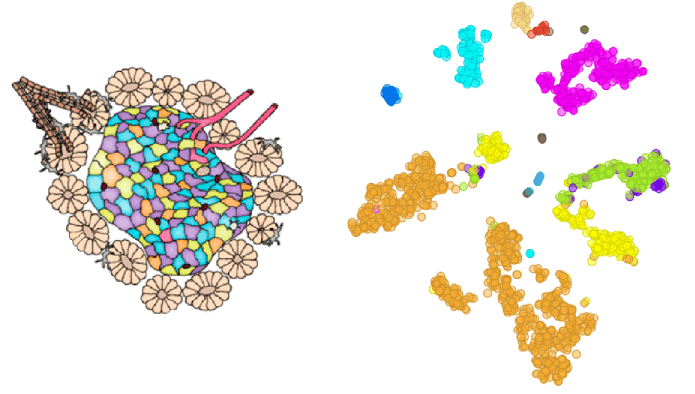